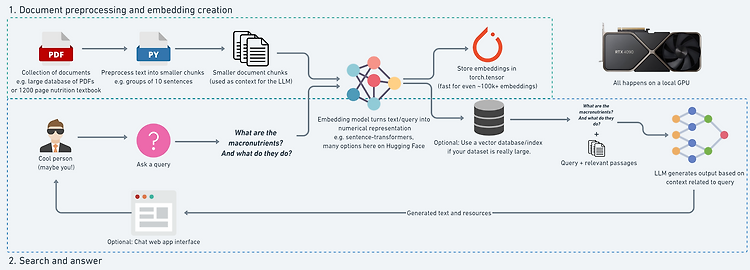
저번 게시글 '처음부터 RAG pipeline 구현하기 - (1)'은 위의 그림에서 [1] 문서 전처리 및 Embedding 생성 단계 에 대해서 다뤄봤다면, 이번 게시글은 Retriever, Augmentation, Generation 과정을 구체적으로 다뤄볼 예정이다.RAG - Search and AnswerRetrieval: 주어진 query와 관련된 resource 가져오기Augmentation: 관련 resource를 prompt에 입력하여, LLM이 해당 정보를 기반으로 텍스트 생성Generation: 검색된 resource를 활용하여 강화(증강)된 응답 생성Similarity searchImport embeddings & embedding modelimport randomimport torc..